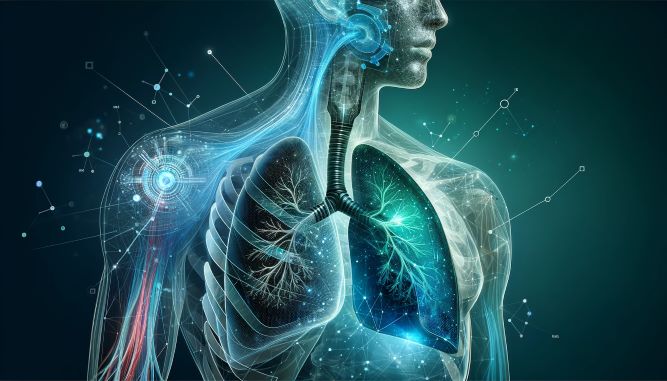
Introduction: The Pneumonia Challenge
Pneumonia remains one of the most significant health challenges globally, being a primary cause of hospitalization and leading to severe respiratory failure. Traditional tools for assessing the severity of pneumonia, such as the Pneumonia Severity Index (PSI), primarily focus on mortality risks. However, these tools often fall short in predicting the need for advanced respiratory support, leaving a crucial gap in patient care.
The novel study led by Yewande E. Odeyemi and her team marks a significant leap in addressing this gap. Their research, titled “Early Machine Learning Prediction of Hospitalized Patients at Low Risk of Respiratory Deterioration or Mortality in Community-Acquired Pneumonia: Derivation and Validation of a Multivariable Model,” introduces a novel approach using machine learning to enhance pneumonia prognosis. This method not only predicts mortality risk but also the likelihood of needing advanced respiratory support.
A New Approach in Pneumonia Prognosis
The research team’s innovative methodology employs a gradient boosting machine (GBM) learning method. This sophisticated technique analyzes a vast range of variables, including patient demographics, vital signs, and laboratory results, all collected within the first six hours of hospital admission. The resulting model offers a more accurate and comprehensive risk assessment, potentially revolutionizing how we approach pneumonia treatment.
The Significance of Community-Acquired Pneumonia (CAP)
Community-Acquired Pneumonia (CAP) is a prevalent and potentially serious infectious disease, characterized by the development of lung infections in individuals who have not recently been hospitalized or had regular exposure to healthcare settings. It is distinguished from hospital-acquired or healthcare-associated pneumonia, which often involves different pathogens and resistance patterns. CAP’s diagnosis and treatment are particularly challenging due to the variability in pathogens and affected patient populations. This variability underscores the importance of accurate and early prognosis for effective treatment.
Machine learning, a branch of artificial intelligence, involves using algorithms and statistical models to enable computers to perform tasks based on patterns and inference. In healthcare, machine learning can process vast amounts of data, identifying patterns not immediately apparent to humans. This ability is particularly beneficial in disease prognosis, treatment personalization, and outcome prediction.
Results: A New Benchmark in Prediction
The study’s approach involved analyzing data from a substantial cohort of 4,379 patients hospitalized with CAP over a ten-year period (2009-2019). The GBM model’s performance was compared against traditional tools like PSI and CURB-65. This large sample size provided a rich dataset, essential for the development and validation of the machine learning model. Patients with various comorbidities were included, as these are common in CAP and can significantly affect its prognosis. Comprehensive lab tests, within the first six hours of admission, including blood cell counts, renal function tests, and markers of infection, were analyzed. These provide vital information for diagnosing pneumonia and assessing its severity. Chest radiography is a standard diagnostic tool for CAP, used to confirm the presence of an infection in the lung.
The study revealed that the machine learning model outperformed traditional tools, offering a C-statistic of 0.71, indicating higher sensitivity (72%) and a negative predictive value of approximately 85%. This accuracy is crucial for informed decision-making in patient care, particularly regarding the need for advanced respiratory support.
This innovative approach paves the way for a future where healthcare is more personalized and efficient. Accurately predicting a patient’s need for advanced respiratory support or mortality risk can significantly improve healthcare resource allocation and pneumonia management.
Nonetheless, integrating machine learning into clinical practice presents challenges, including data privacy, ethical implications, and algorithmic bias. Addressing these challenges requires ongoing research and collaboration among medical professionals, data scientists, and ethicists.
The study by Odeyemi and colleagues highlights the potential of machine learning in revolutionizing healthcare. It offers a more precise tool for pneumonia prognosis and serves as a blueprint for AI integration across various medical fields. Embracing technological advancements will be key to developing a more efficient, effective, and personalized healthcare system.
Reference: Odeyemi YE, Lal A, Barreto EF, LeMahieu AM, Yadav H, Gajic O, Schulte P. Early machine learning prediction of hospitalized patients at low risk of respiratory deterioration or mortality in community-acquired pneumonia: Derivation and validation of a multivariable model. Biomol Biomed [Internet]. 2023Oct.4 [cited 2024Jan.17];. Available from: https://www.bjbms.org/ojs/index.php/bjbms/article/view/9754
Editor: Ermina Vukalic
Leave a Reply